Remote Sensing and its future?
Remote Sensing and its future?
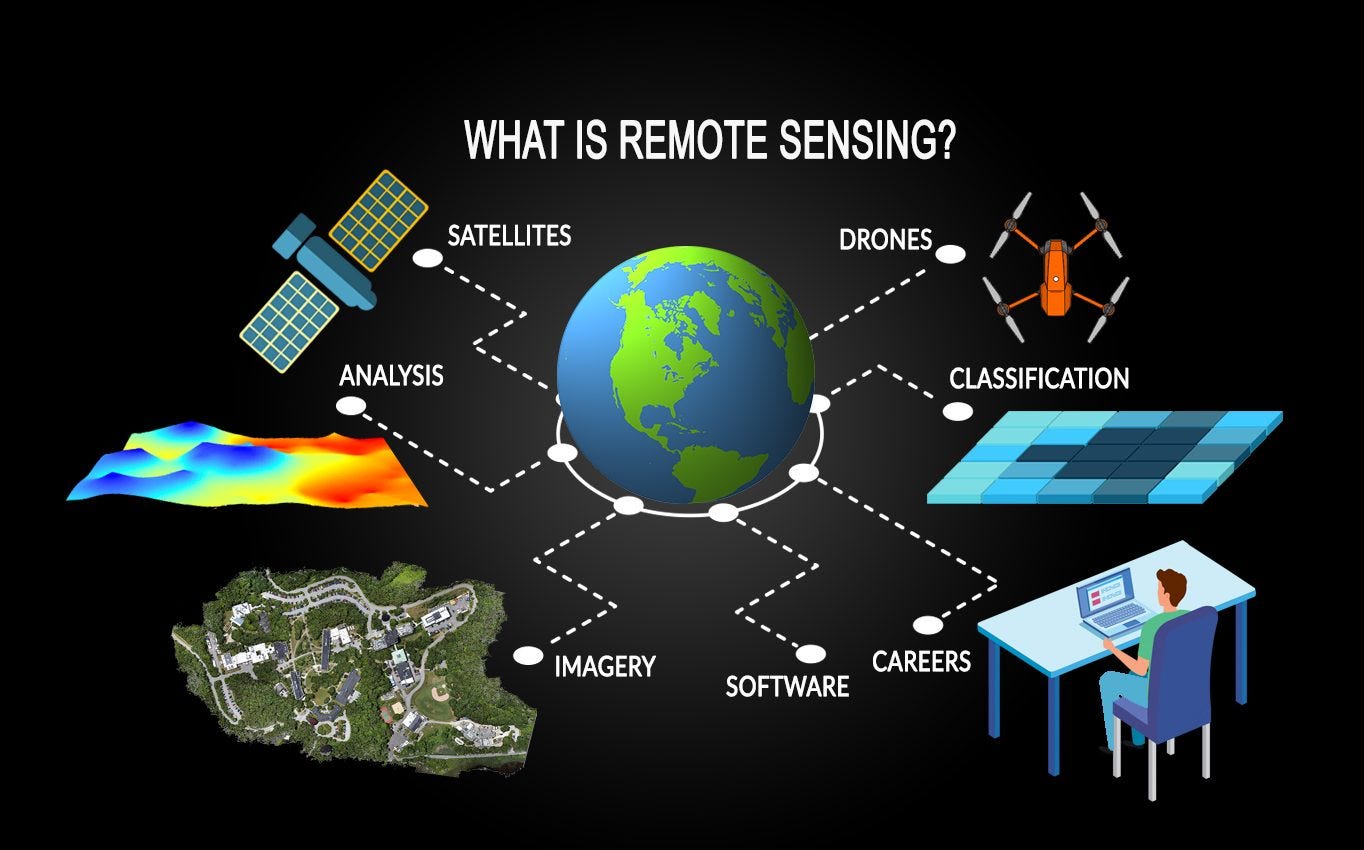
Remote Sensing
If you guys are reading this then you would have come across this term while reading about climate change or something to do with satellite image processing in various domains. Many of you would have given a pass to this term and wouldn’t have bothered to understand the broad meaning of it. But as remotely sensed images are becoming more common and are being used in many analytical data stack there is a need to understand this term.
According to NOAA : Remote sensing is the science of obtaining information about objects or areas from a distance, typically from aircraft or satellites.
Remote sensing in itself has been a hot topic for research for a long time and still is, it was present before the inception of Deep Learning. Then the Data was coarser in resolution (Spatial, Temporal & Radiometric) and was limited to only few use cases.
At present, remote sensing is being perceived in a very different way than what it use to be before. This change is due to availability of varied open source satellite data and high resolution satellite imagery surfacing in open datasets. Moreover new research integrating deep learning with these datasets have opened up a plethora of use cases in tech as well as in business world.
It is also considered to be the heart of satellite image data processing. Science of remote sensing is actually about how information is captured, stored and how we can generate insights from it.
Is this similar to Image processing/computer vision?
No, But yes we do apply image processing on remotely sensed images. Also using satellite imagery with only the 3 bands RGB will not take you anywhere.
Many computer vision engineer ignore the fact that aerial images have more than 3 bands and they often ignore spatial context of data.
Remote sensing images requires to be handled differently. Compare it this way, in finance domain we do feature modelling for our datasets that add value to the model we create. Similarly, to create valuable features from satellite imagery we need to apply remote sensing knowledge.
If you want to learn more about Remote Sensing click here.
Current Trends & Challenges
1. Synthetic Aperture Radar (SAR)
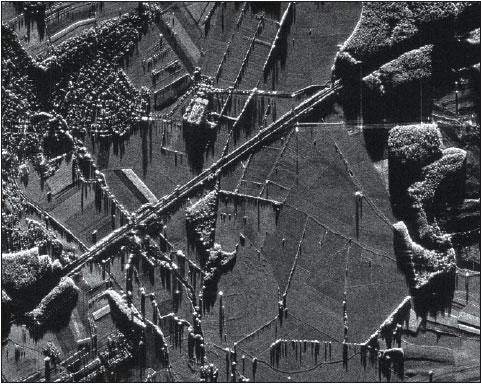
SAR has been present in the remote sensing stack since the inception of remote sensing. Although its use was to map the earth surface below and above sea and also in defence sector. Since the release of Sentinel-1 imagery product for free and thus research in SAR has shown a positive rise. It is being used in agriculture, mining, oil and gas, water resources and many such setores. The major reason why SAR is promising because the way it is captured and how you can create many different intermediate imagery from Level 1 product.
I won’t be covering about SAR imagery here, will leave it for another day as it is in itself a very interesting topic to dive in.
2. Deep Learning & Remote Sensing
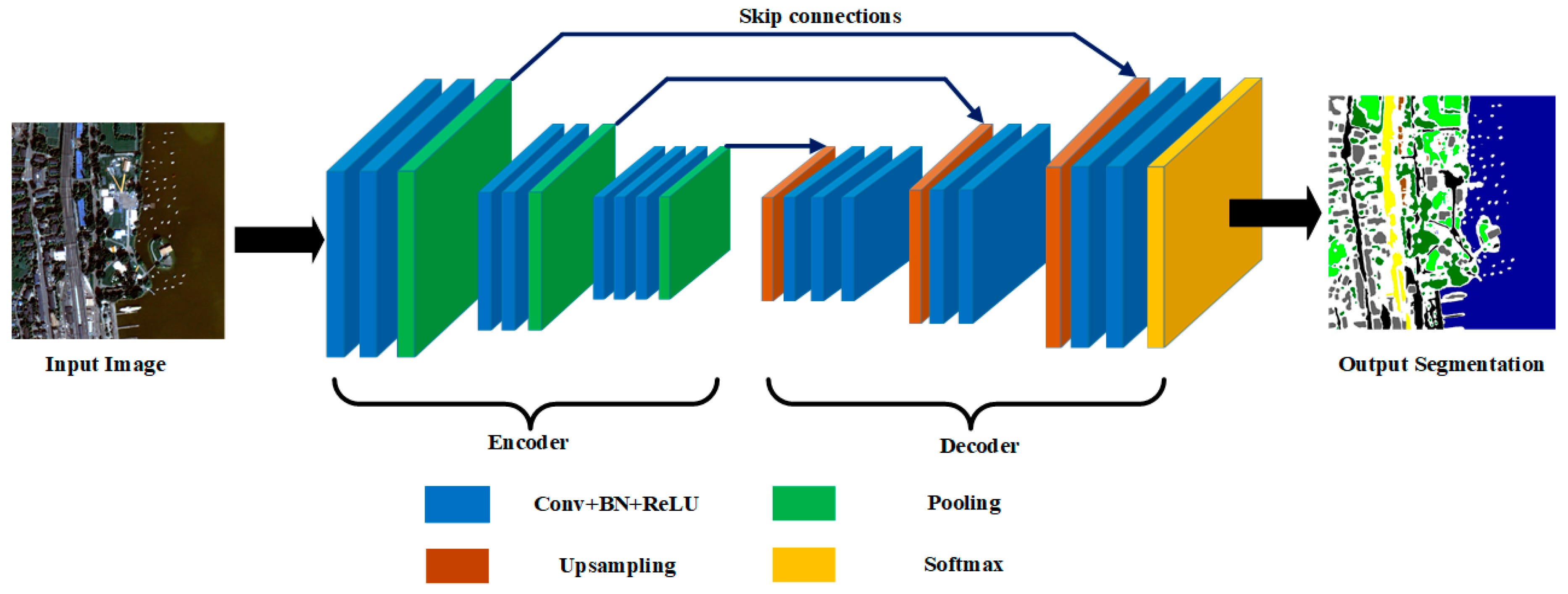
Satellite Image Segmentation using U-net Arch (source)
Deep Learning has already found its place in aerial imagery. Many computer vision experts have exploited RGB bands of satellite imagery for segmentation and pattern detection using high resolution imagery. But when we move into solving more critical problems we need understanding of different bands along side RGB bands. Although I have seen people integrating SAR imagery and Multi spectral imagery together but they are still lacking use of remote sensing information.
One issue that I have noticed here is if you are creating a DL model using a test data for a city like Brussels the same model would fail for a city like California. Thus we would require a separate training sets for each city/country.
To have a successful Deep learning model people should not be using aerial imagery from the shelf. Instead they need to create various intermediate indices and features for input in their deep learning model.
You guys can check success of U-net architecture in field of Remote Sensing. Also, do check use of GANs in Satellite images.
3. Other Trends
- Agriculture Tech has been on rise for quite a few year, but people are stuck on very basic indices like NDVI, LAI and few more. These indices gives you a broad idea of your overall field conditions but not good enough to be used into for end to end scalable model. It might give you a short term solution but a long lasting solution needs to integrate other components as well.
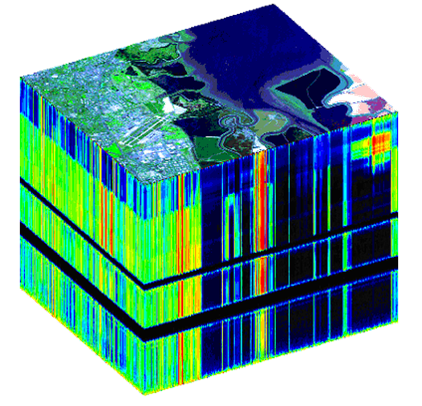
Hyper-spectral Image having more than 20 bands
- Hyper spectral imagery is also one of the trends which is catching up. Although we don’t have enough data for open source community to exploit it, but mineral explorers are always on watch for this data.
- It wouldn't be fair to not mention about climate change and environment studies and there are already many research papers around it. Aerosols monitoring, Nitrogen dioxide content in air, Glacier study and many such studies are happening and have already happened in remote sensing domain. There are few new startups coming in the field of climate change where they are trying to figure out how various business entities can have a impact on climate change and how we can have a work around it without affecting your current business profits.
- Do check Google Earth Engine (GEE) various datasets available for use for free and how you can leverage GEE + Colab for ML and DL models. This is picking up pace from past few months.
Future for Remote Sensing ?
Remote sensing is going to be mainstream aspect of many business solution in coming future. I say this because privatisation of space industry has opened all new Space Tech market. Check YourStory for more current trends around Space Tech. These space tech would be an aggregation of firms having their own rockets, own satellite clusters and will be having access to high res imagery of whole earth capturing from different sensors. Building a next level platform integrating different business solution with high resolution satellite imagery is something I can’t wait to see. Also, Remote sensing will flourish alongside the space tech market as well as in research works.